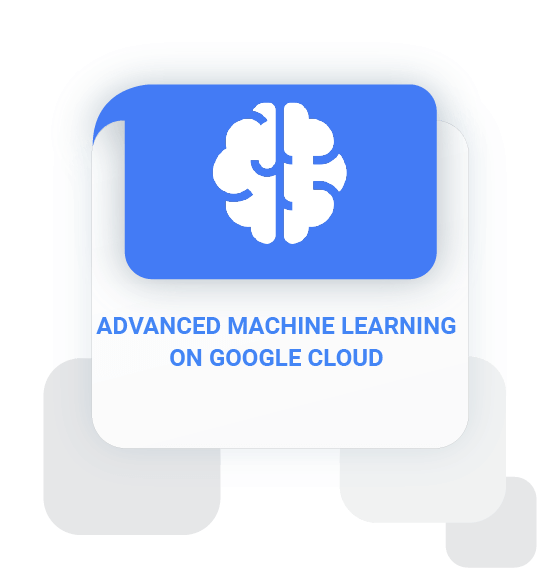
Tổng quan khoá học
Khóa học giúp bạn có thực hành việc triển khai, tối ưu và mở rộng các model ML ứng dụng vào thực tế. Bạn sẽ học được cách xây dựng các mô hình có khả năng tự mở rộng với độ chính xác và tính ứng dụng thực tế cao với nhiều loại dữ liệu như dữ liệu có cấu trúc, hình ảnh, time-series, natural language, cũng như biết cách xây dựng hệ thống recommendation.
Thời gian: 40 giờ
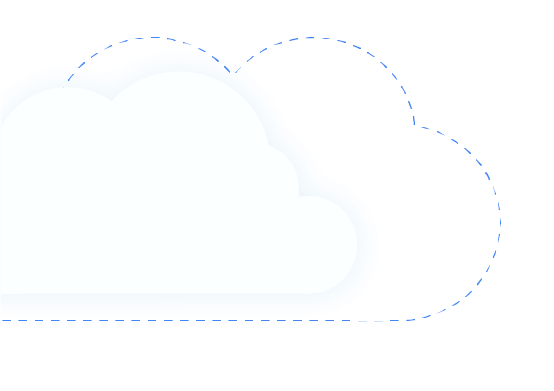
Mục tiêu khóa học
Sau khi học xong khóa học, học viên sẽ nắm được các kiến thức như sau:
- Triển khai nhiều hệ thống ML khác nhau: static/dynamic/continuous training, static/dynamic inference, batching/online processing.
- Giải quyết một vấn đề ML hoàn chình bằng cách xây dựng end-to-end pipeline: khai phá dữ liệu, xử lý & chuẩn bị dữ liệu, lựa chọn thuộc tính, xây dựng model, hiệu chỉnh tham số và triển khai thực tế.
- Xây dựng các mô hình image classification từ các model linear đơn giản đến các mạng hiệu suất cao như CNNs bằng cách thực hiện các kỹ thuật batch normalization, augmentation và transfer learning.
- Forecast dữ liệu time-series sử dụng CNNs, RNNs và LSTMs.
- Áp dụng ML trong việc xử lý ngôn ngữ tự nhiên bằng CNNs, RNNs, LSTMs.
- Triển khai content-based, collaborative, hybrid và neural recommendation model trên TensorFlow.
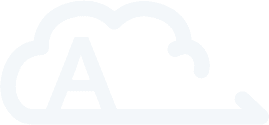
Đối tượng học viên
- Data Engineer hoặc lập trình viên quan tâm đến việc áp dụng Machine learning trong thực tế .
- Cá nhân hứng thú với ứng dụng Machine learning đẻ giải quyết các vấn đề trong kinh doanh cho doanh nghiệp.
Yêu cầu học viên
- Hoàn thành khóa học Big Data & Machine Learning Fundamentals
- Có kiến thức về ML, TensorFlow
- Có kinh nghiệm lập trình Python
- Có kiến thức cơ bản về thống kê
- Có kiến thức cơ bản về SQL
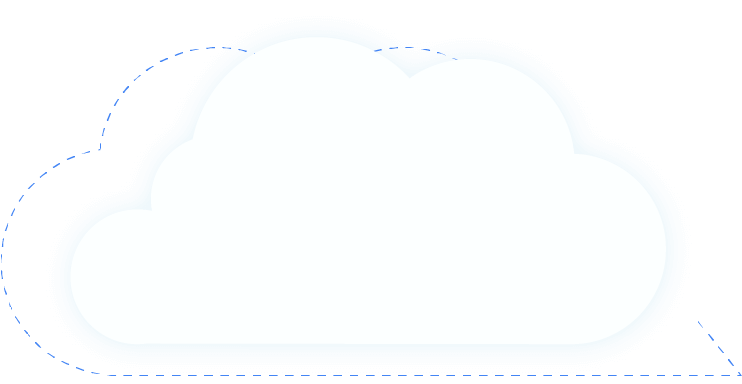
Nội dung khoá học
01
Machine Learning on Google Cloud Platform
Effective ML.
Fully managed ML.
02
Explore the Data
Exploring the dataset.
BigQuery.
BigQuery and AI Platform Notebooks.
03
Creating the dataset
Creating a dataset.
04
Build the Model
Build the model.
05
Operationalize the model
Operationalizing the model.
Cloud AI Platform.
Train and deploy with Cloud AI Platform.
BigQuery ML.
Deploying and Predicting with Cloud AI Platform.
06
Architecting Production ML Systems
The Components of an ML System.
The Components of an ML System: Data Analysis and Validation.
The Components of an ML System: Data Transformation + Trainer.
The Components of an ML System: Tuner + Model Evaluation and Validation.
The Components of an ML System: Serving.
The Components of an ML System: Orchestration + Workflow.
The Components of an ML System: Integrated Frontend + Storage.
Training Design Decisions.
Serving Design Decisions.
Designing from Scratch.
07
Ingesting data for Cloud-based analytics and ML
Data On-Premise.
Large Datasets.
Data on Other Clouds.
Existing Databases.
08
Designing Adaptable ML systems
Adapting to Data.
Changing Distributions.
Right and Wrong Decisions.
System Failure.
Mitigating Training-Serving Skew through Design.
Debugging a Production Model.
09
Designing High-performance ML systems
Training.
Predictions.
Why distributed training?
Distributed training architectures.
Faster input pipelines.
Native TensorFlow Operations.
TensorFlow Records.
Parallel pipelines.
Data parallelism with All Reduce.
Parameter Server Approach.
Inference.L
10
Hybrid ML systemsL
Machine Learning on Hybrid Cloud.
KubeFlow.
Embedded Models.
TensorFlow Lite.
Optimizing for Mobile.
11
Welcome to Image Understanding with TensorFlow on GCP
Images as Visual Data.
Structured vs Unstructured Data.
12
Linear and DNN Models
Linear Models.
DNN Models Review.
Review: What is Dropout
13
Convolutional Neural Networks (CNNs)
Understanding Convolutions.
CNN Model Parameters.
Working with Pooling Layers.
Implementing CNNs with TensorFlow.
14
Dealing with Data Scarcity
The Data Scarcity Problem.
Data Augmentation.
Transfer Learning.
No Data, No Problem.
15
Going Deeper Faster
Batch Normalization.
Residual Networks.
Accelerators (CPU vs GPU, TPU).
TPU Estimator.
Neural Architecture Search.
16
Pre-built ML Models for Image Classification
Pre-built ML Models.
Cloud Vision API.
AutoML Vision.
AutoML Architecture.
17
Working with Sequences
Sequence data and models.
From sequences to inputs.
Modeling sequences with linear models.
Modeling sequences with DNNs.
Modeling sequences with CNNs.
The variable-length problem
18
Recurrent Neural Networks
Introducing Recurrent Neural Networks.
How RNNs represent the past.
The limits of what RNNs can represent.
The vanishing gradient problem.
19
Dealing with Longer Sequences
LSTMs and GRUs.
RNNs in TensorFlow.
Deep RNNs.
Improving our Loss Function.
Working with Real Data.
20
Text Classification
Working with Text.
Text Classification.
Selecting a Model.
Python vs Native TensorFlow.
21
Reusable Embeddings
Historical methods of making word embeddings.
Modern methods of making word embeddings.
Introducing TensorFlow Hub.
Using TensorFlow Hub within an estimator.
22
Recurrent Neural NetworksEncoder-Decoder Models
Introducing Encoder-Decoder Networks.
Attention Networks.
Training Encoder-Decoder Models with TensorFlow.
Introducing Tensor2Tensor.
AutoML Translation.
Dialogflow.
23
Module 23: Recommendation Systems Overview
Types of Recommendation Systems.
Content-Based or Collaborative.
Recommendation System Pitfalls.
24
Content-Based Recommendation Systems
Content-Based Recommendation Systems.
Similarity Measures.
Building a User Vector.
Making Recommendations Using a User Vector.
Making Recommendations for Many Users.
Using Neural Networks for Content-Based Recommendation Systems.
25
Collaborative Filtering Recommendation Systems
Types of User Feedback Data.
Embedding Users and Items.
Factorization Approaches.
The ALS Algorithm.
Preparing Input Data for ALS.
Creating Sparse Tensors For Efficient WALS Input.
Instantiating a WALS Estimator: From Input to Estimator.
Instantiating a WAL Estimator: Decoding TFRecords.
Instantiating a WALS Estimator: Recovering Keys.
Instantiating a WALS Estimator: Training and Prediction.
Issues with Collaborative Filtering.
Cold Starts.
26
Neural Networks for Recommendation Systems
Hybrid Recommendation System.
Context-Aware Recommendation Systems.
Context-Aware Algorithms.
Contextual Postfiltering.
Modeling Using Context-Aware Algorithms.
27
Building an End-to-End Recommendation System
Architecture Overview..
Cloud Composer Overview.
Cloud Composer: DAGs.
Cloud Composer: Operators for ML9.
Cloud Composer: Scheduling.
Cloud Composer: Triggering Workflows with Cloud Functions.
Cloud Composer: Monitoring and Logging.
Học cùng các
chuyên gia Goole Cloud
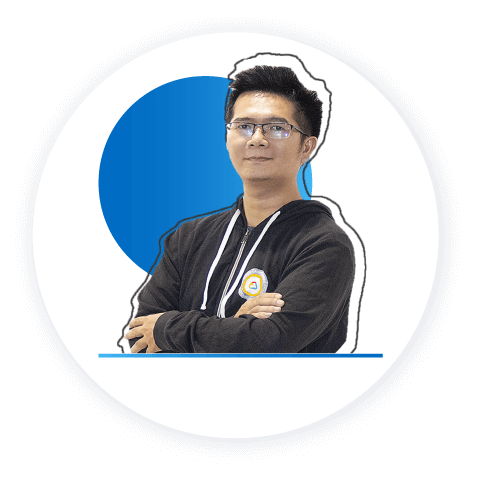
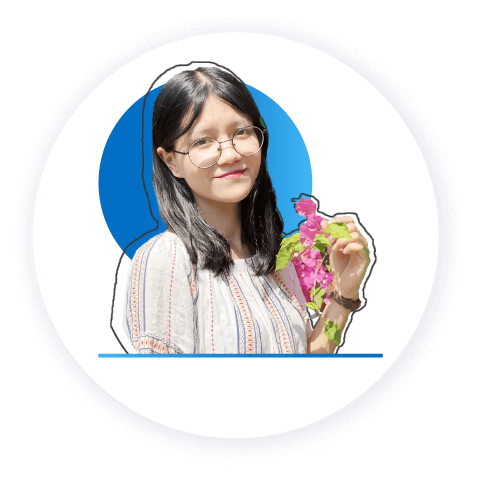
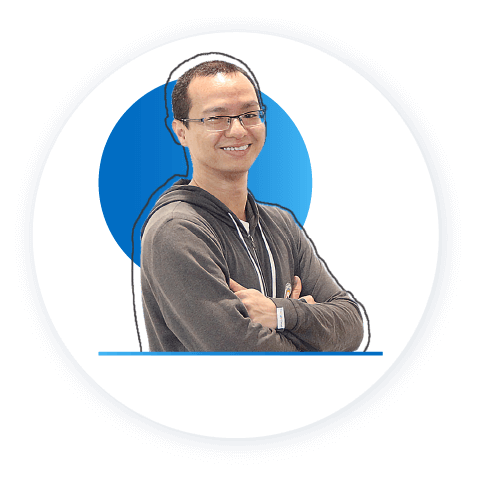
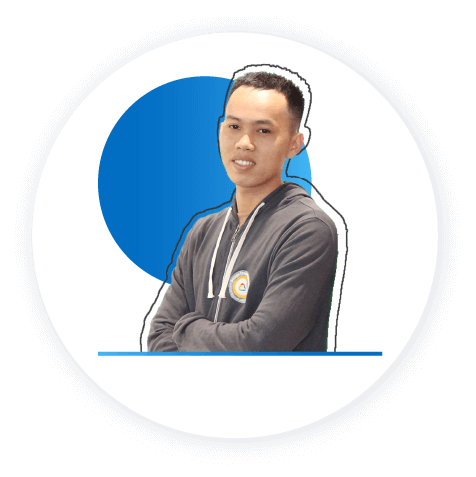
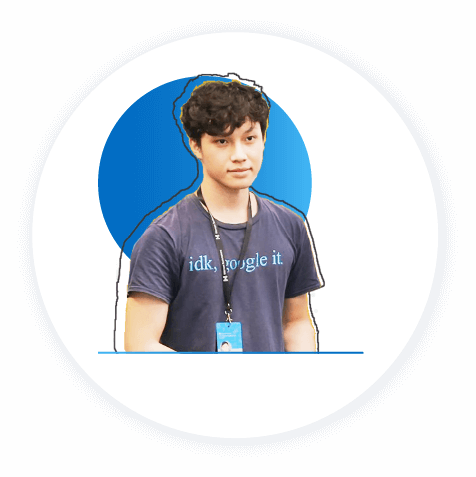
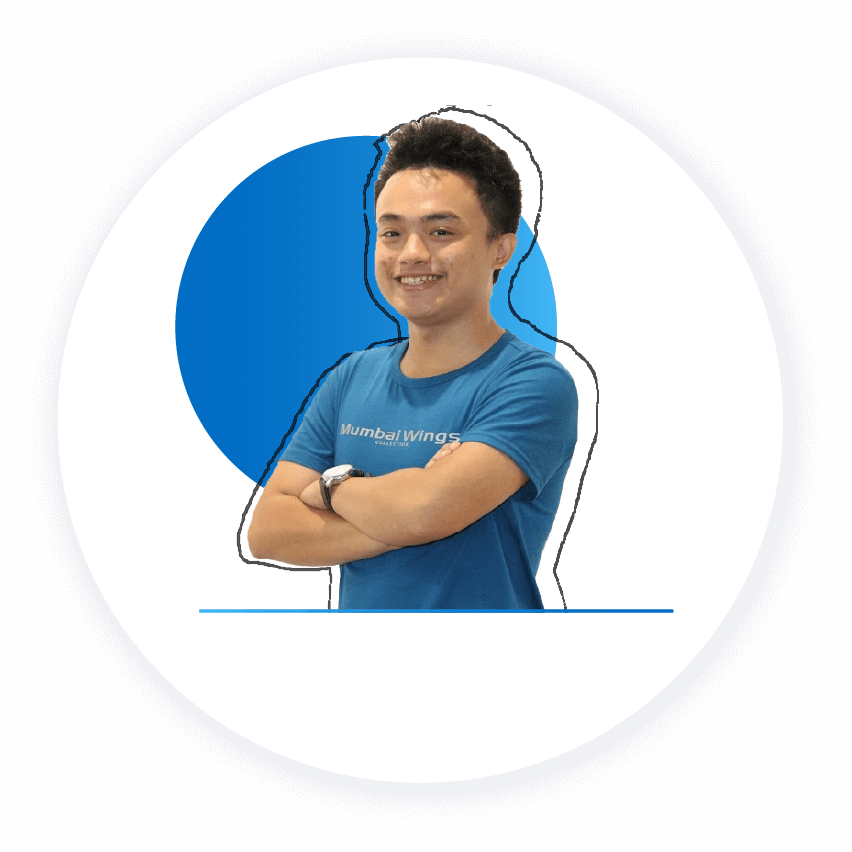
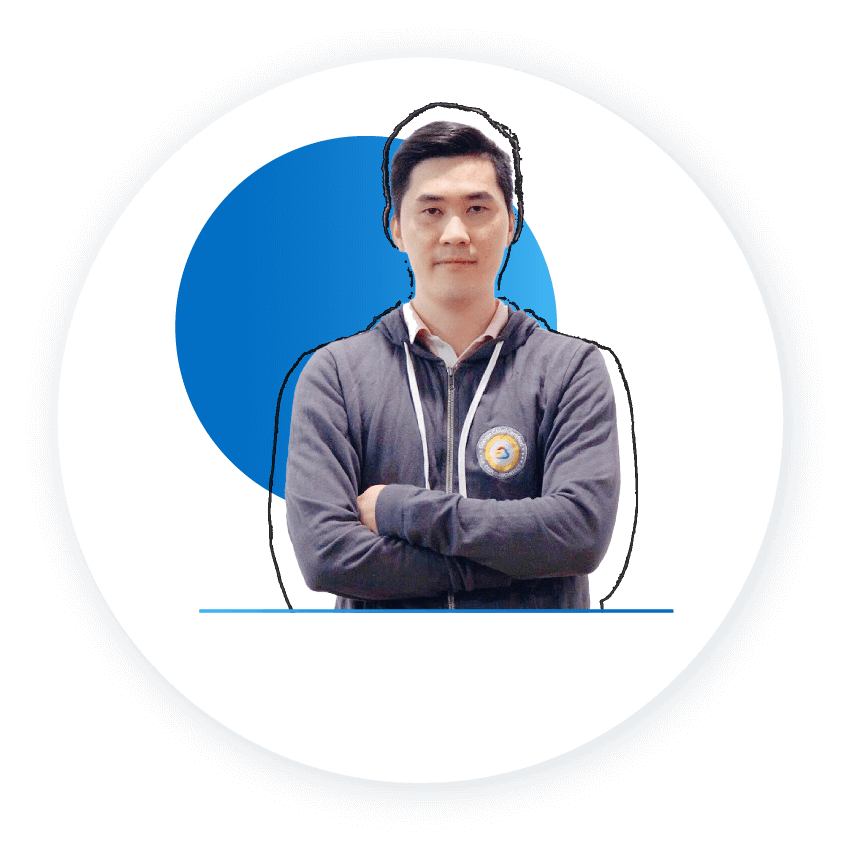
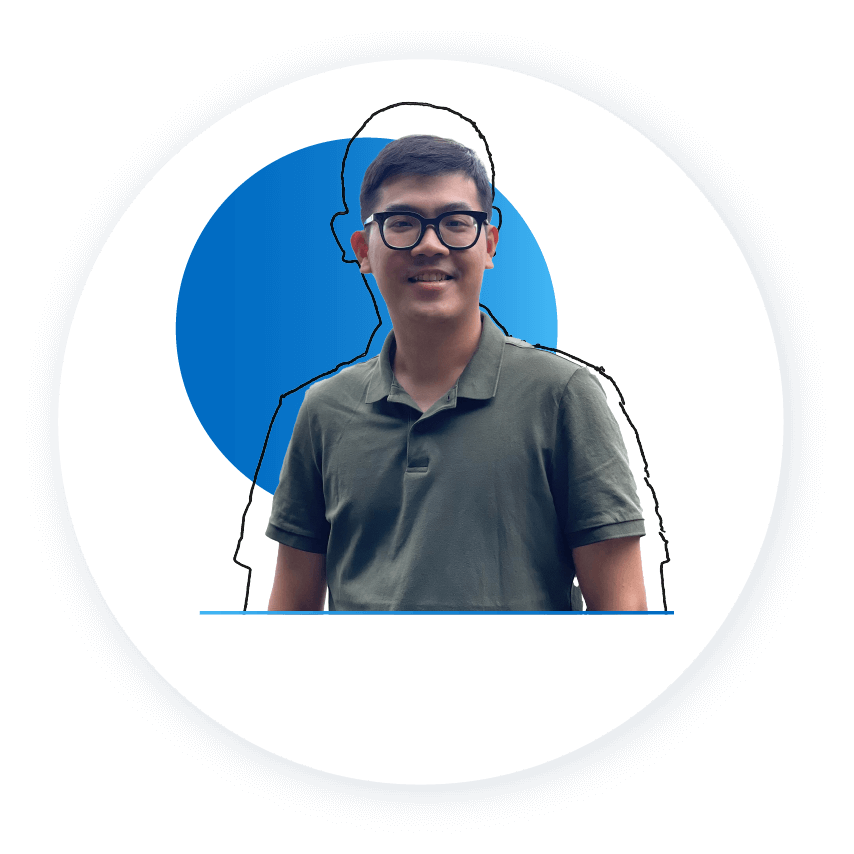
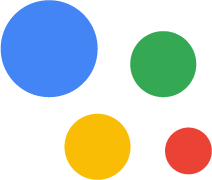
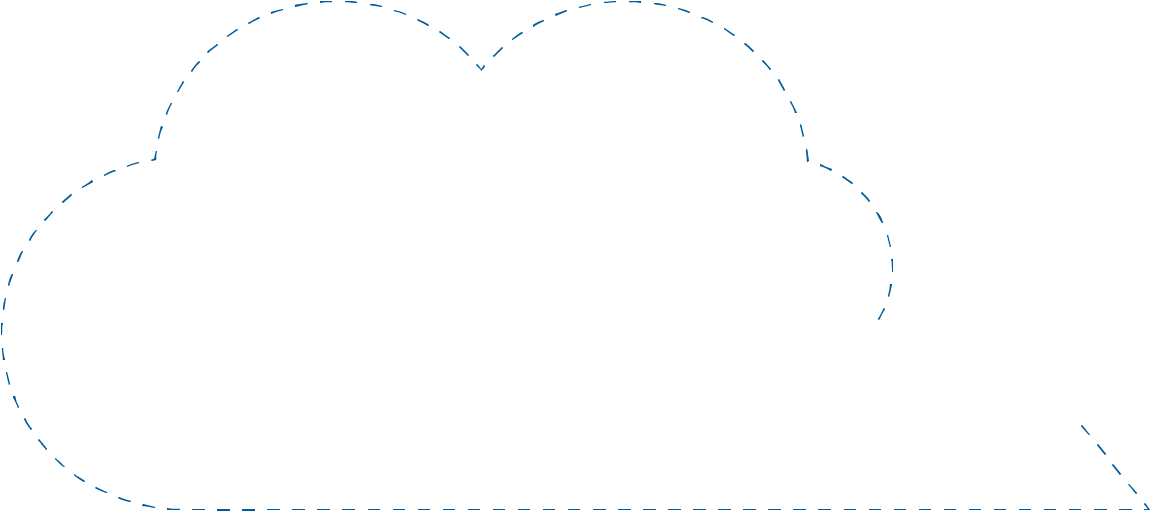
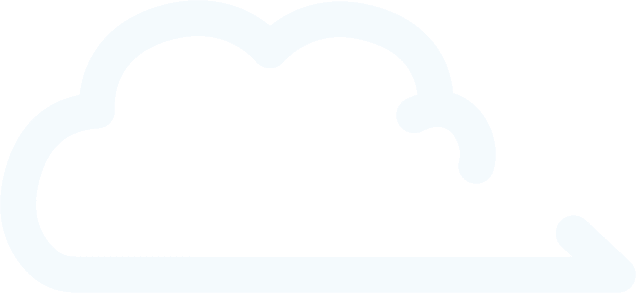
Cảm nghĩ học viên
Cloud Ace Training
đem đến những trải nghiệm tuyệt vời cho học viên
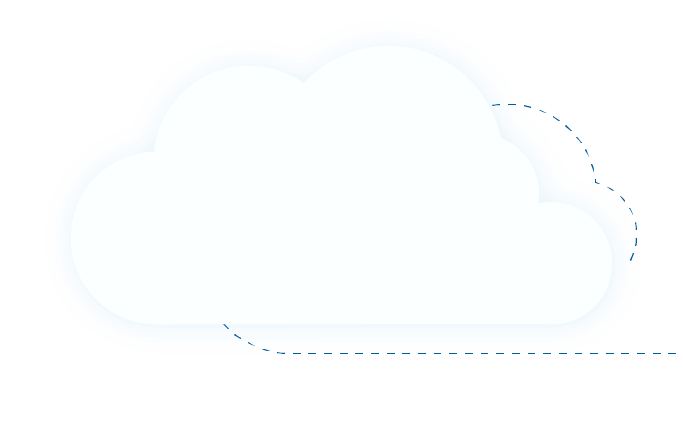

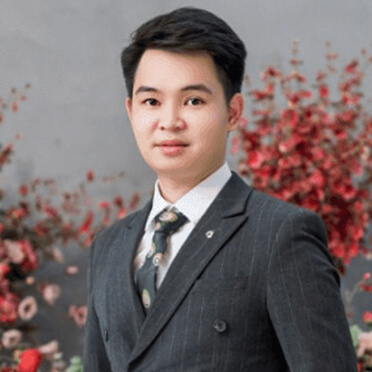
Trần Tuấn Anh
IT

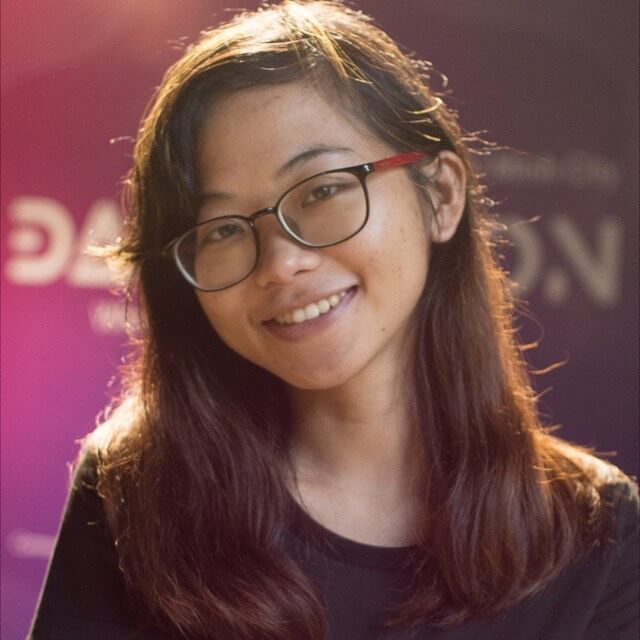
Nguyễn Ngọc Minh Thy
Data Engineer

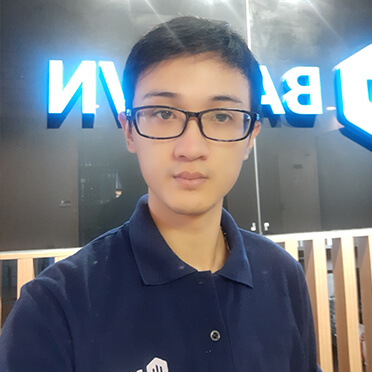
Trương Quốc Thắng
Data Engineer


Phạm Văn Hùng
IT

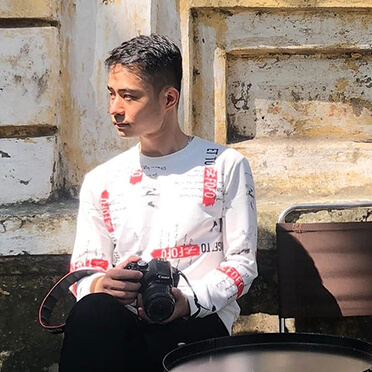
Dương Minh Phương
Engineer
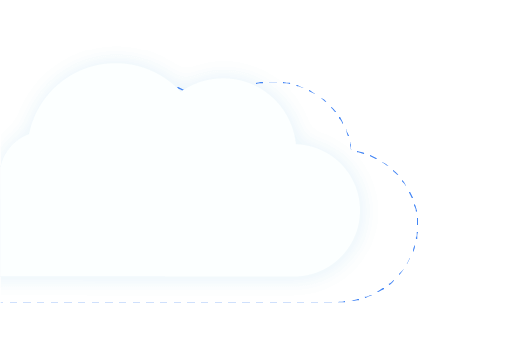
Đăng ký ngay để trở thành
" Chuyên gia Google Cloud "
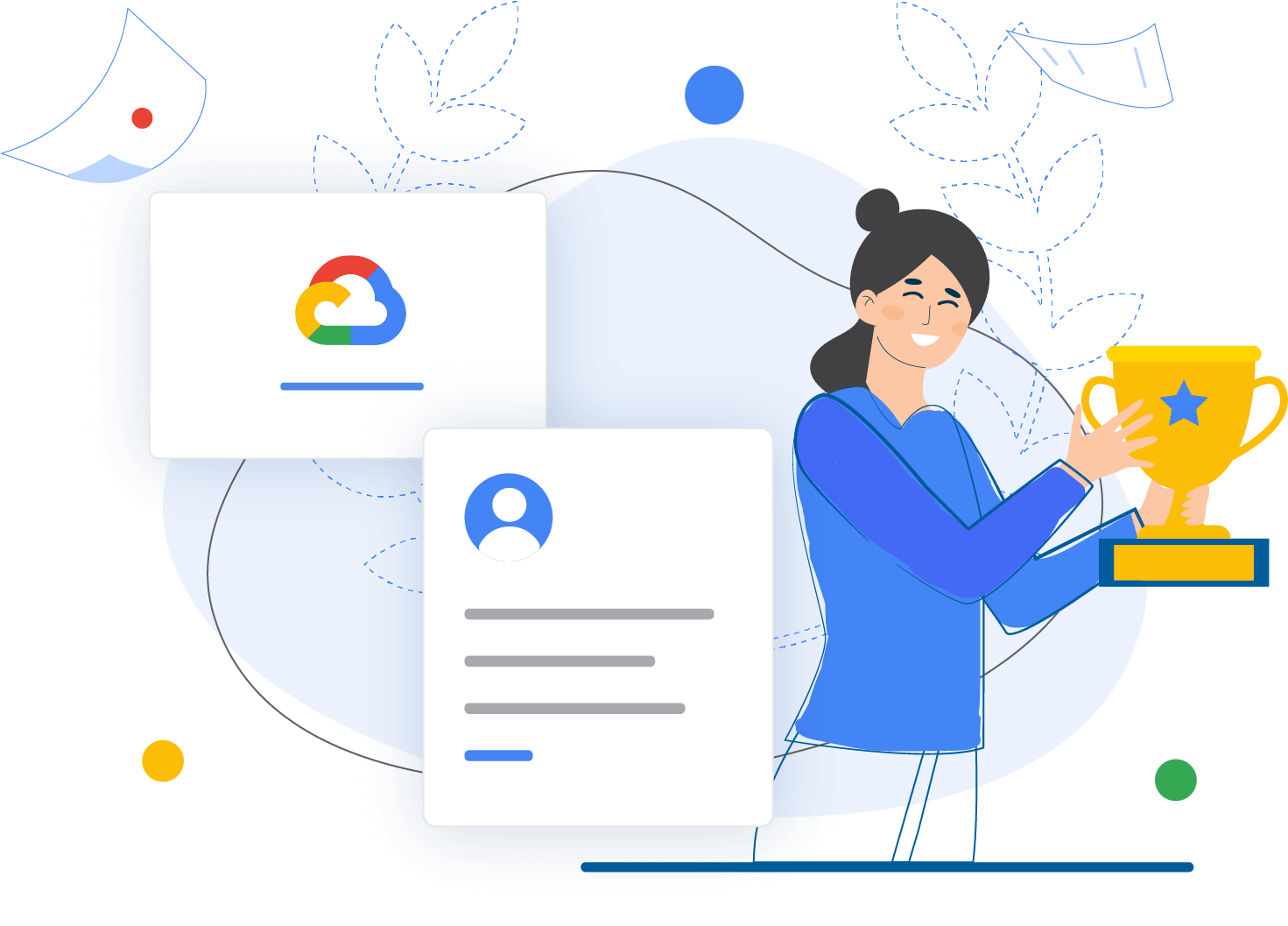
câu hỏi thường gặp
Cloud Ace là đơn vị đào tạo Google Cloud nên không tổ chức thi và cung cấp chứng chỉ Google Cloud. Cloud Ace chỉ hỗ trợ cung cấp chứng nhận hoàn thành khóa học cho học viên trong quá trình đợi thi lấy chứng Google Cloud
Ngoài ra, nếu bạn muốn thi lấy chứng chỉ Google Cloud, thì Cloud Ace sẽ hướng dẫn đăng ký thi Online hoặc Offline tại các trung tâm khảo thí ủy quyền của Google Cloud tại Việt Nam
Dĩ nhiên là được, trong suốt quá trình học, bạn sẽ liên tục được giải các bài Quiz, các bài thi thử mô phỏng gần giống với đề thi thực tế của Google Cloud. Ngoài ra, Cloud Ace còn cung cấp các câu hỏi Dump liên tục cập nhật các dạng đề, câu hỏi thi từ Google Cloud giúp bạn có sự chuẩn bị tốt nhất cho kỳ thi.
Tất nhiên là có rồi. bạn sẽ được Cloud Ace hỗ trợ trong quá trình học và kể cả khi kết thúc khóa học. Bạn có thể tương tác với Trainer qua Slack, email hoặc qua Group Google Cloud Plartform User HCM để được các Trainer hỗ trợ nhé.
Sau khi học xong khóa học, nếu bạn có thắc mắc nào về phần kiến thức hoặc gặp khó khăn trong quá trình triển khai dự án trên Google Cloud thì có thể liên hệ với Trainer để được giải đáp thắc mắc nhé.
Khóa học Google Cloud không chỉ phù hợp với các kỹ sư phần mềm hay kỹ sư phát triển hệ thống mà còn phù hợp với các kỹ sư xử lý dữ liệu như Data Engineer, Data Scientist.
Ngoài ra, nếu bạn đang là Marketer hay làm việc trong lĩnh vực tài chính, ngân hàng, TMDT, Logistics…. liên tục phải đối mặt với dữ liệu lớn cần giải quyết thì có thể tham khảo các khóa học Big Data Machine Learning Fundamental hoặc các khóa From Data to Insight on Google Cloud Plartform để tham khảo các xử lý dữ liệu đơn giản và tạo báo cáo chuyên nghiệp trên Google Cloud nhé.